
Building an
AI-Native Startup
Founder Survey 2024
What is the real impact of GenAI on the startup ecosystem? This summer, Costanoa surveyed 30 founders about their experiences building in the AI space, 21 of whom built their companies within the last two years. Here are some of the insights into how the startup landscape is evolving alongside GenAI.
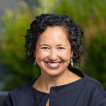
Martina Lauchengco
Partner,
Costanoa Ventures

Amanda Paolino
Investment Fellow,
Costanoa Ventures
Key Findings
Talent.
Cofounders with an AI-background aren’t considered necessary,
but they do impact early hiring decisions.
- Nearly two-thirds of all respondents do not believe they need an AI cofounder.
- Companies with an AI cofounder are 47% more likely to hire an engineer with academic or AI research experience than those without (90% vs 61%).
- Nearly 54% of companies prioritize go-to-market roles for early hires.
- Repeat founders are 33% more likely to establish their company headquarters in the San Francisco Bay Area than first-time founders (56% vs. 42%).
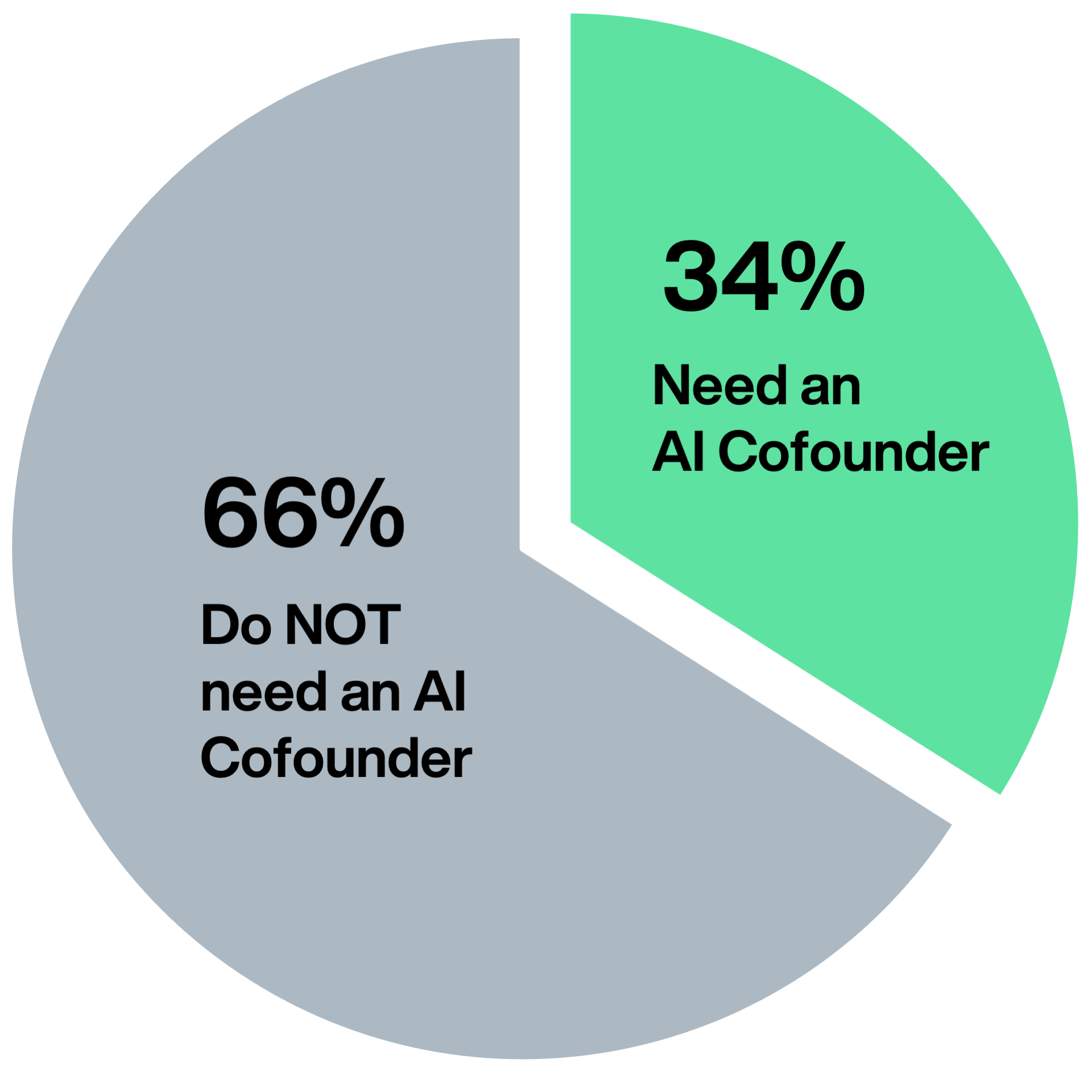
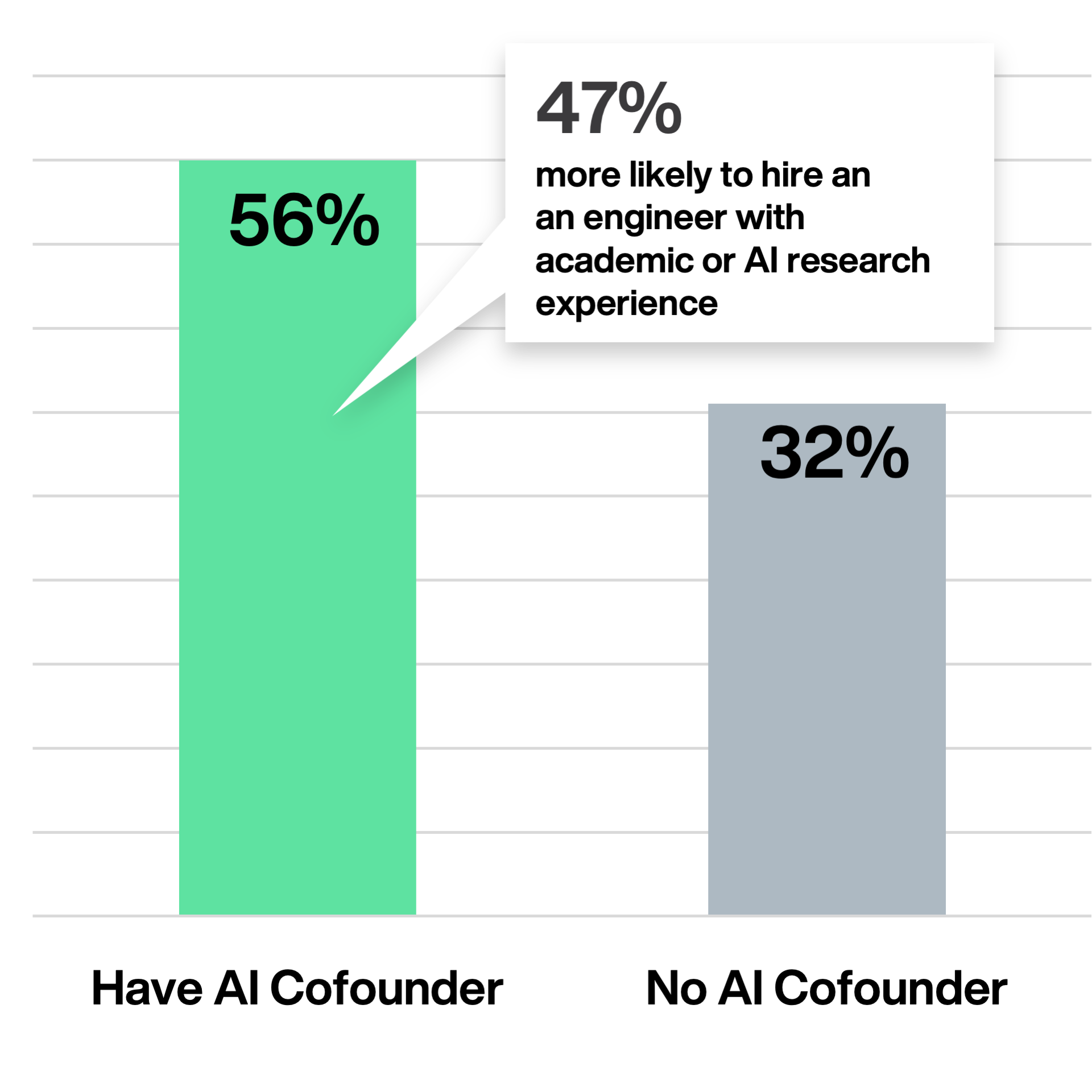
Technology.
While GPT is a mainstay, 75% of respondents use more than one model,
with Gemini, Llama, and Claude gaining popularity.
- Over half (54%) of all respondents are using four or more foundation models.
- Nearly nine in ten founders (89%) are using some version of GPT, followed by Llama and Claude at 40%.
- Pre-seed and seed stage companies are twice as likely to use Mistral as Series A or later companies.
- 44% of repeat founders consider cost a main factor in foundation model selection, compared to just 25% of first-time founders.
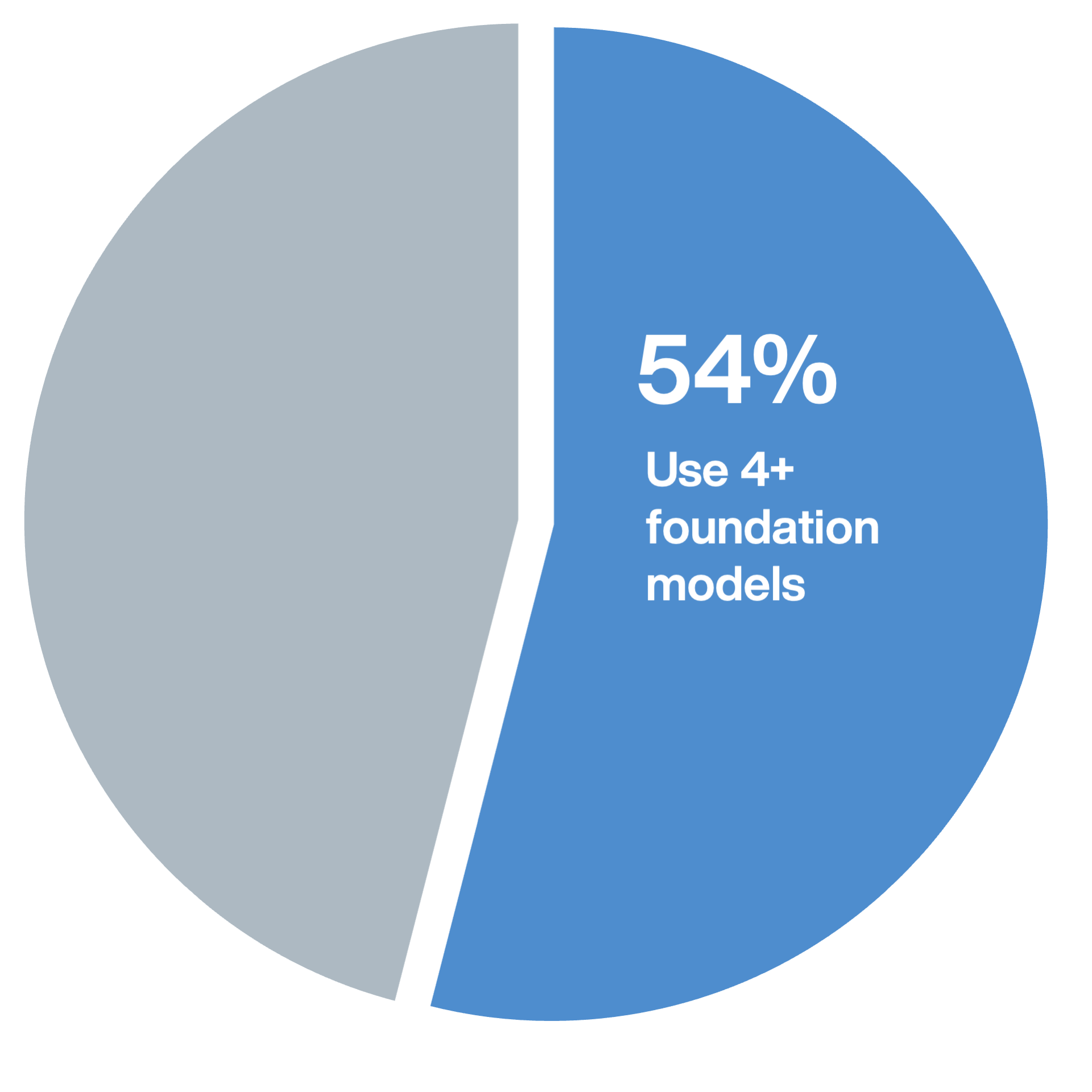
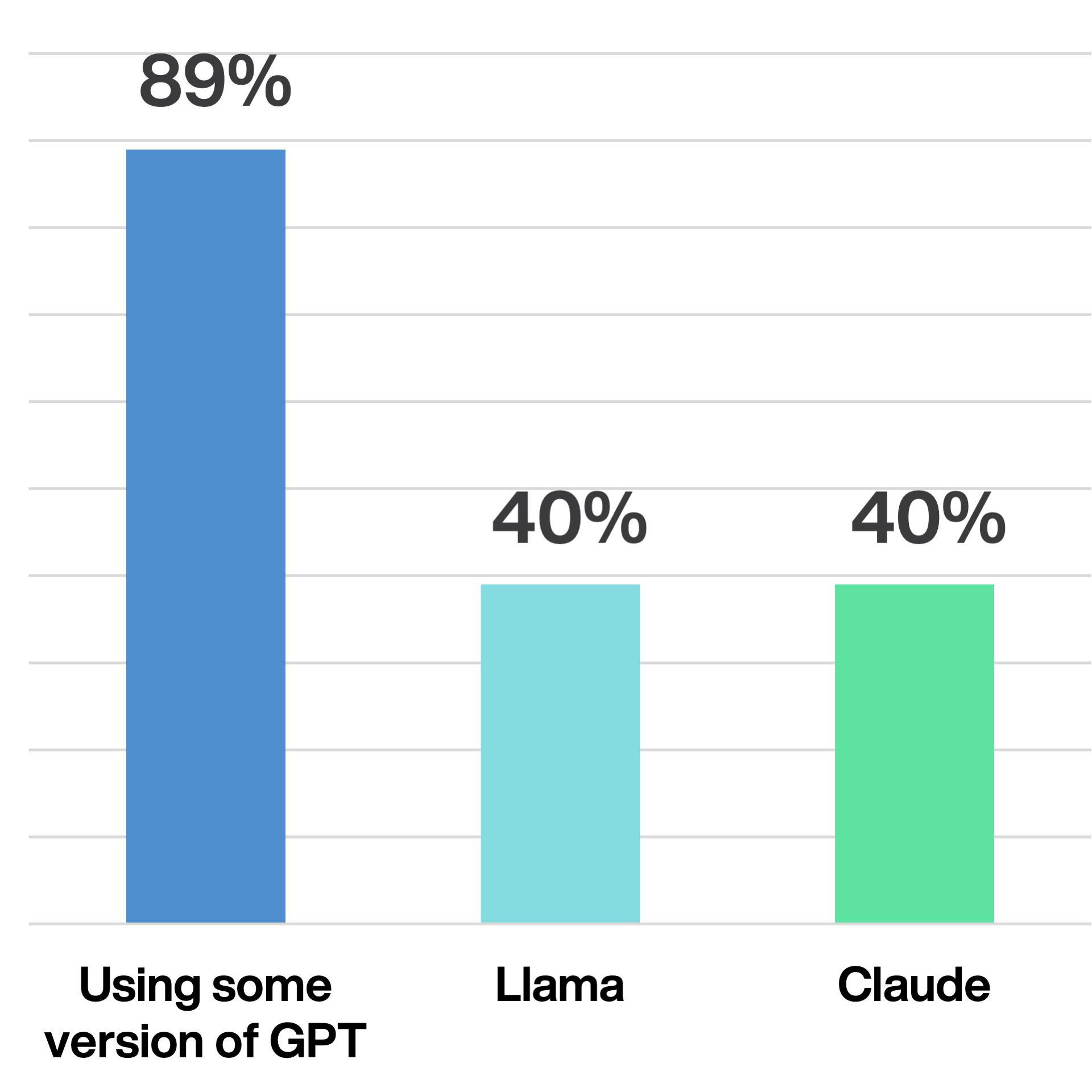
Challenges.
Experienced founders are more attuned to go-to-market and cost considerations.
- Acquiring customers matches hiring talent as the biggest challenges facing founders.
- Repeat founders are twice as likely to mention “noise in the market” as the most painful part of building in AI right now.
- Only 13% of founders express even slight concern about defensibility.
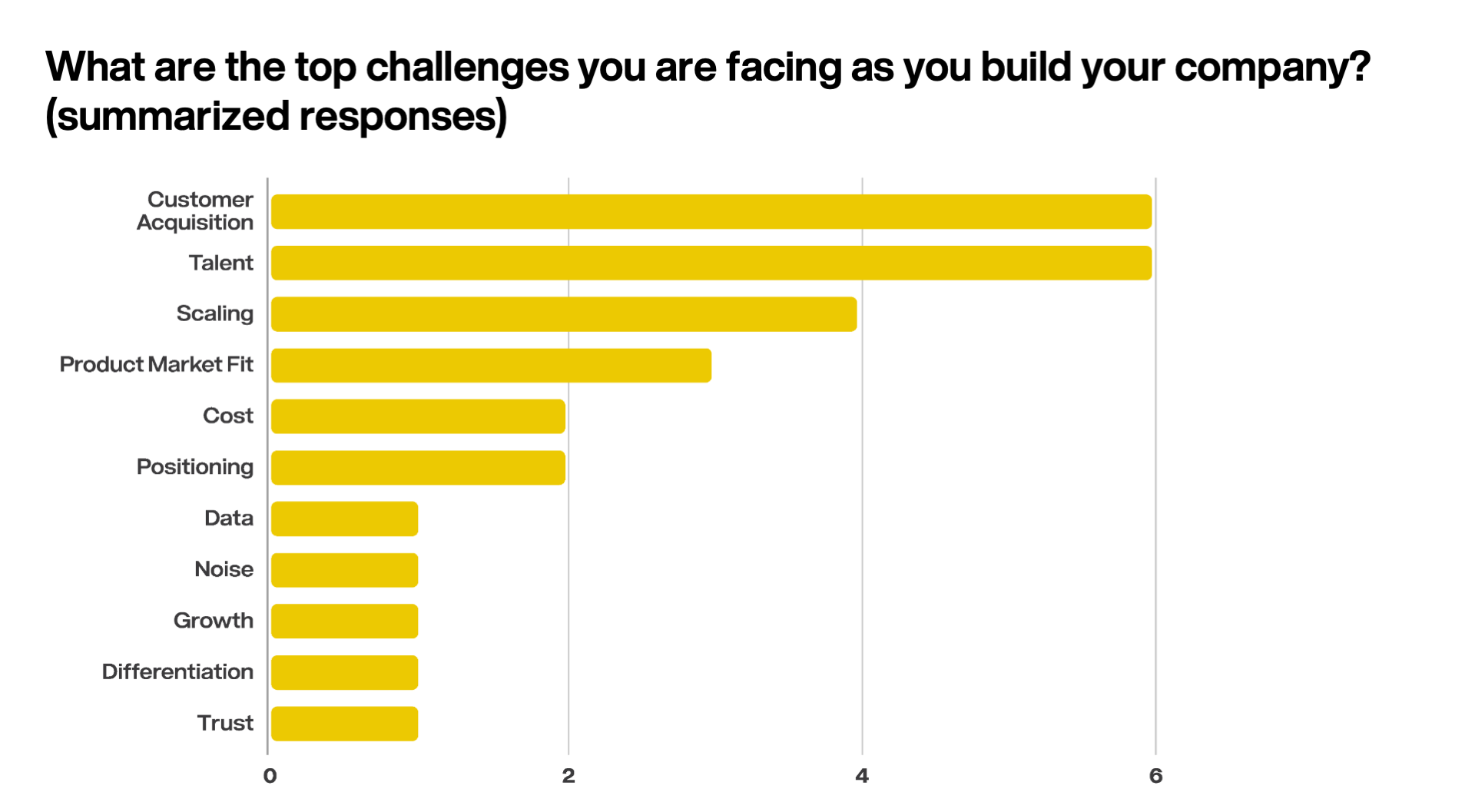
Talent & Culture:
How founders think about hiring and AI expertise
The common wisdom is that hiring at an early stage startup is intensely competitive and difficult, especially for engineering roles — but these AI founders reveal a more nuanced landscape.
Is it important to be headquartered in the San Francisco Bay Area?
The majority of global investment in AI startups happens in Silicon Valley — and half of survey respondents call the Bay Area home. But many others choose alternate geographies, including New York City, Seattle, Washington D.C., and even Lehi, Utah.
- 50% located in the Bay Area
- 36% located elsewhere
- 14% fully remote
That said, second-time founders are 33% more likely to start their company in the Bay Area versus first-timers, citing proximity to talent and investors as a driving force.
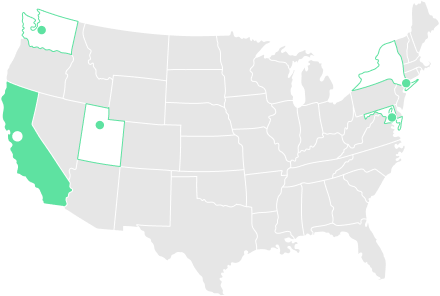
“There is no better place in the world to work on the most ambitious projects of this generation than San Francisco.” —Agent Ops
“New York City has a strong and fast-growing tech ecosystem, lots of talent, and there’s no strong need for us to be in San Francisco since we don’t sell primarily to other startups.” —Cassidy
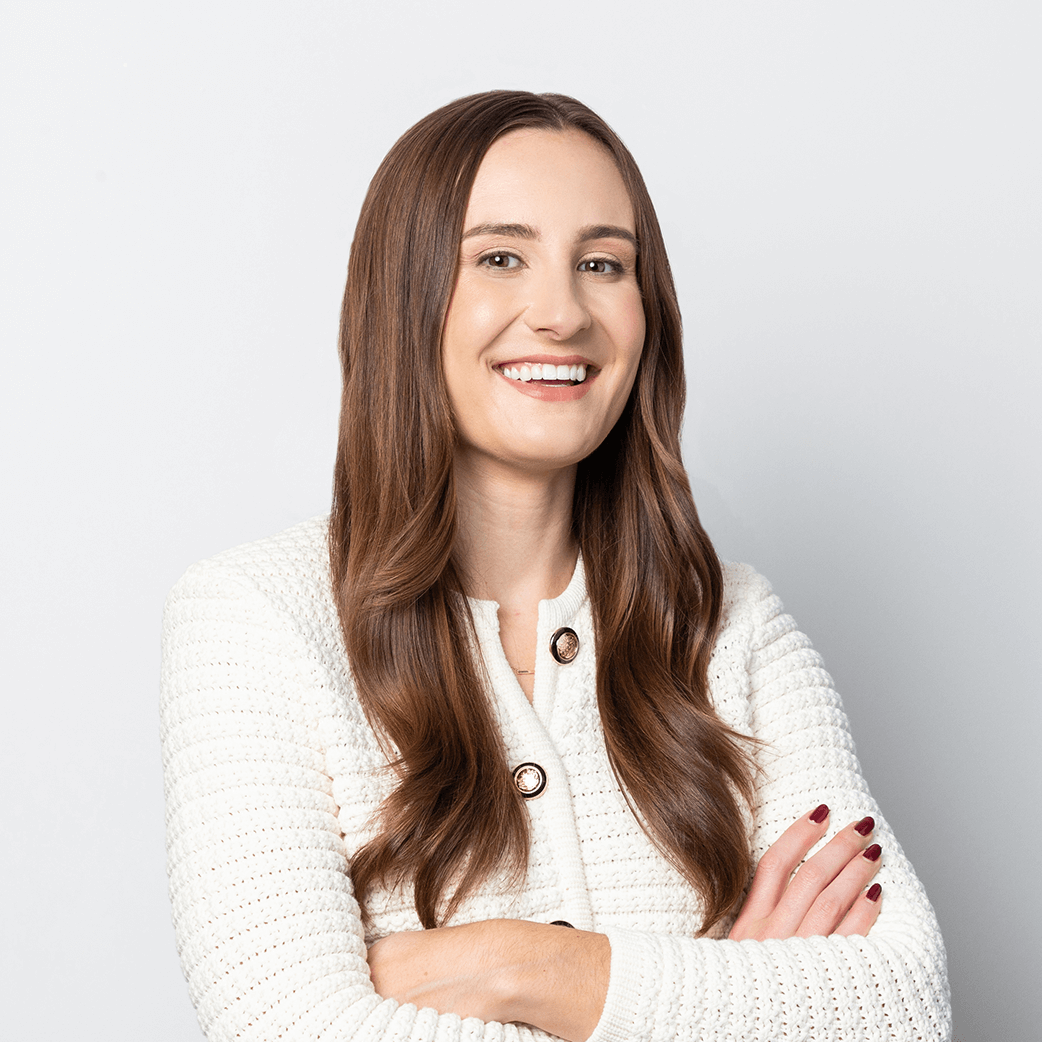
Investor Insight: Amy Cheetham
“It’s interesting that, despite general agreement on talent being more dispersed than ever, more experienced founders are still choosing the Bay Area. There is a bias around talent from this part of the world.”
Is an AI cofounder needed?
There doesn’t appear to be a “one size fits all” model for seeking out an AI cofounder. Just under one-third of companies founded after 2022 note they either have or feel the need for a cofounder with a PhD or background in applied models. Founder history, product type, location — there’s no detectable pattern to the prioritization of an AI cofounder.
Some founders note an AI cofounder helps with product development and fundraising, while others say it’s more important to have this skill set represented on the engineering team.
“Yes, I believe that [my cofounder’s] long history of AI work was hugely beneficial in us taking a clear-eyed view of AI and building a differentiated stack.” —AgentOps
“I don’t feel I need an ‘AI cofounder’ for this work, as the technology approach is more at the systems level, utilizing various AI tools. That said, it’s clear to me that in the not-so-distant future, building up that NLP/computer vision/related skill sets will be important to have on the team.” —Ichi
“I wanted an AI cofounder to ensure we had that kind of DNA in the company, but ultimately went with a traditional SaaS CTO. I kept looking for a ‘cofounder-level’ AI person and ended up bringing one on board.” —Stealth Startup
“Not necessarily. I think the best fit is someone who has range.” —AirMDR
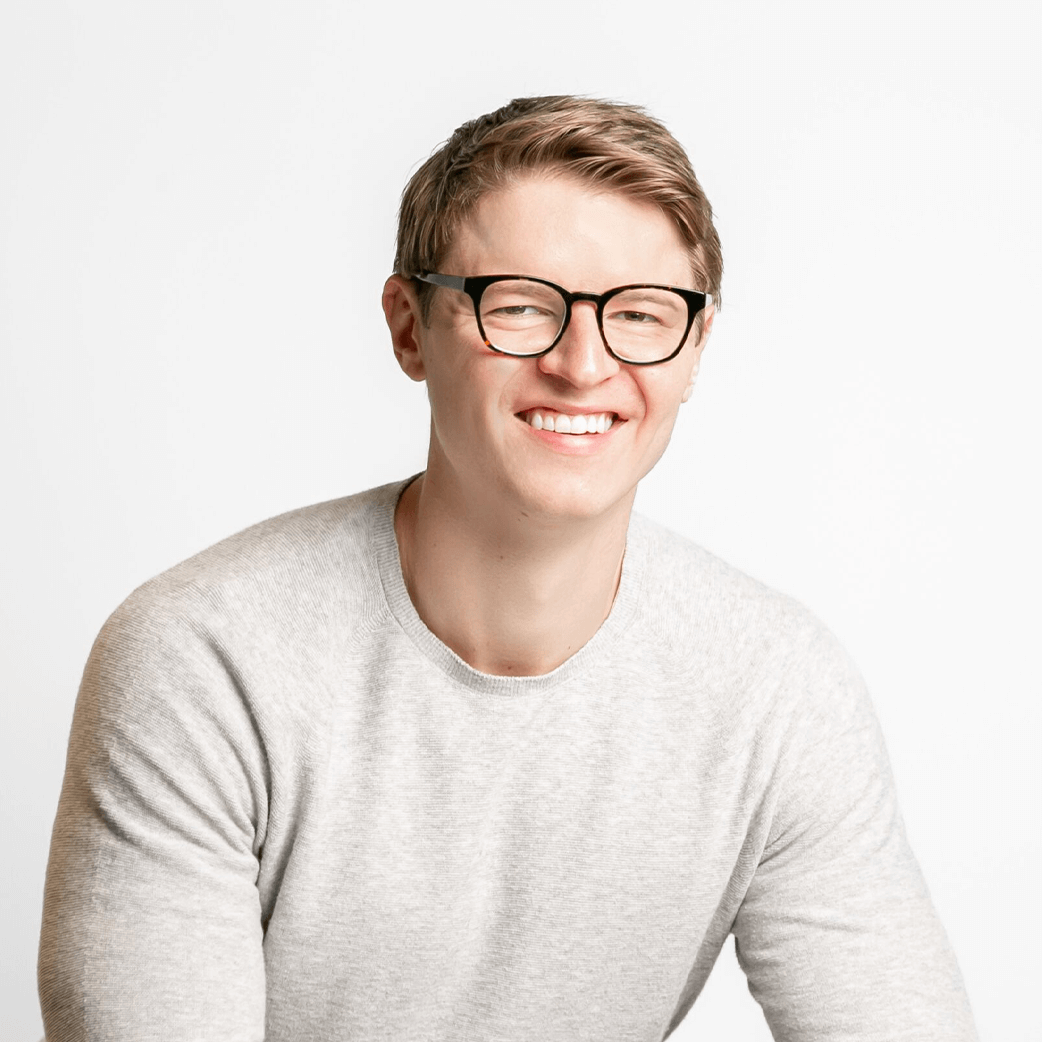
Investor Insight: John Cowgill
“This reaffirmed what we see: the companies getting the most leverage from AI today often aren’t founded by people with an AI PhD or years of experience in AI. They’re made up of entrepreneurial, quick study engineers who have the curiosity and ‘just build’ mentality to keep up with the bleeding edge in AI.”
Which roles get hired first?
Over 70% of startups already have an engineer with an AI research background and/or prompt and applied model experience — but the presence of several key roles vary across stages. The founders’ backgrounds seem to be a considerable factor in talent prioritization. Second-time founders are marginally more likely to hire across all roles, while companies with an AI cofounder are significantly more likely to employ an an engineer with academic or AI research experience — 90% vs. 61%, respectively.
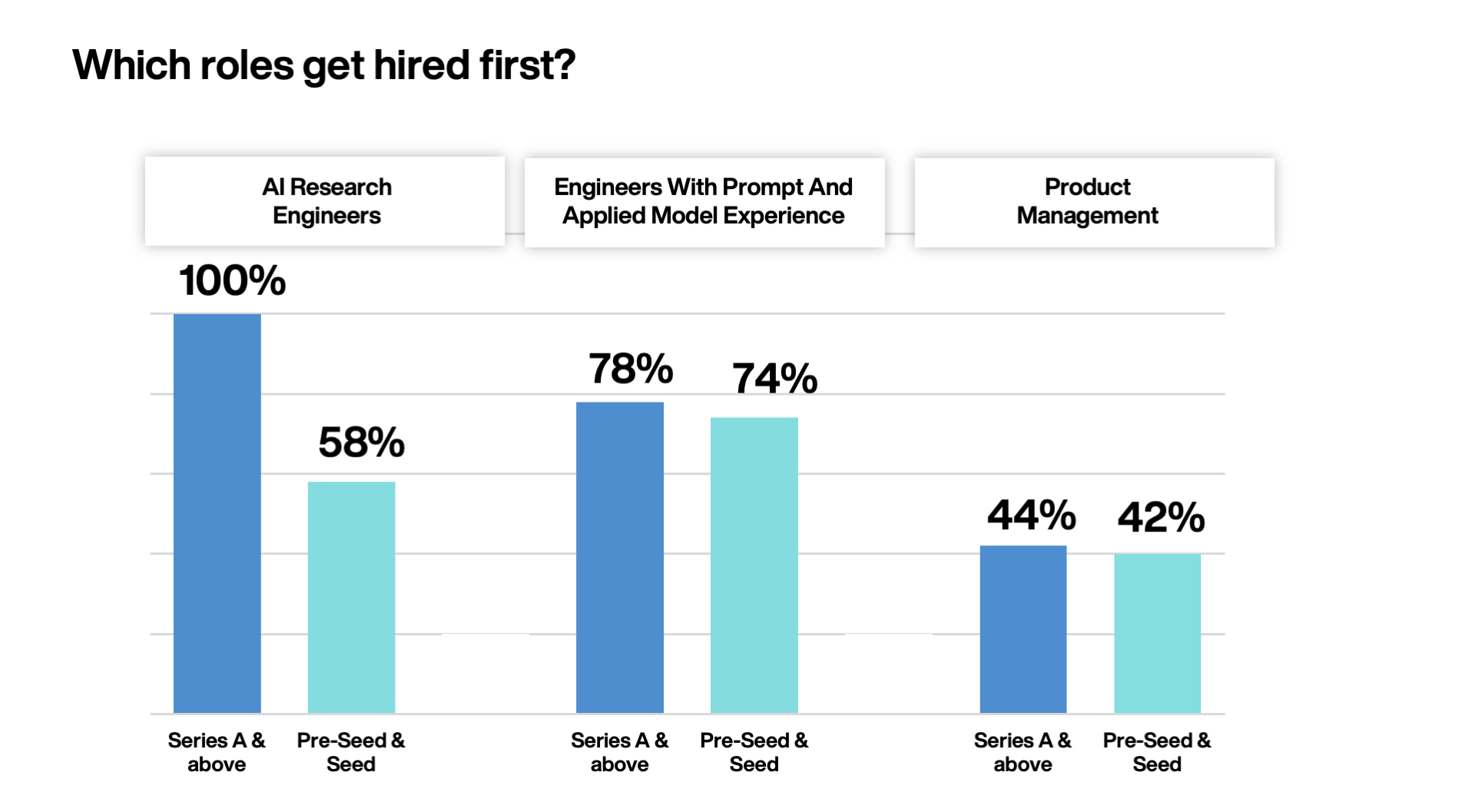
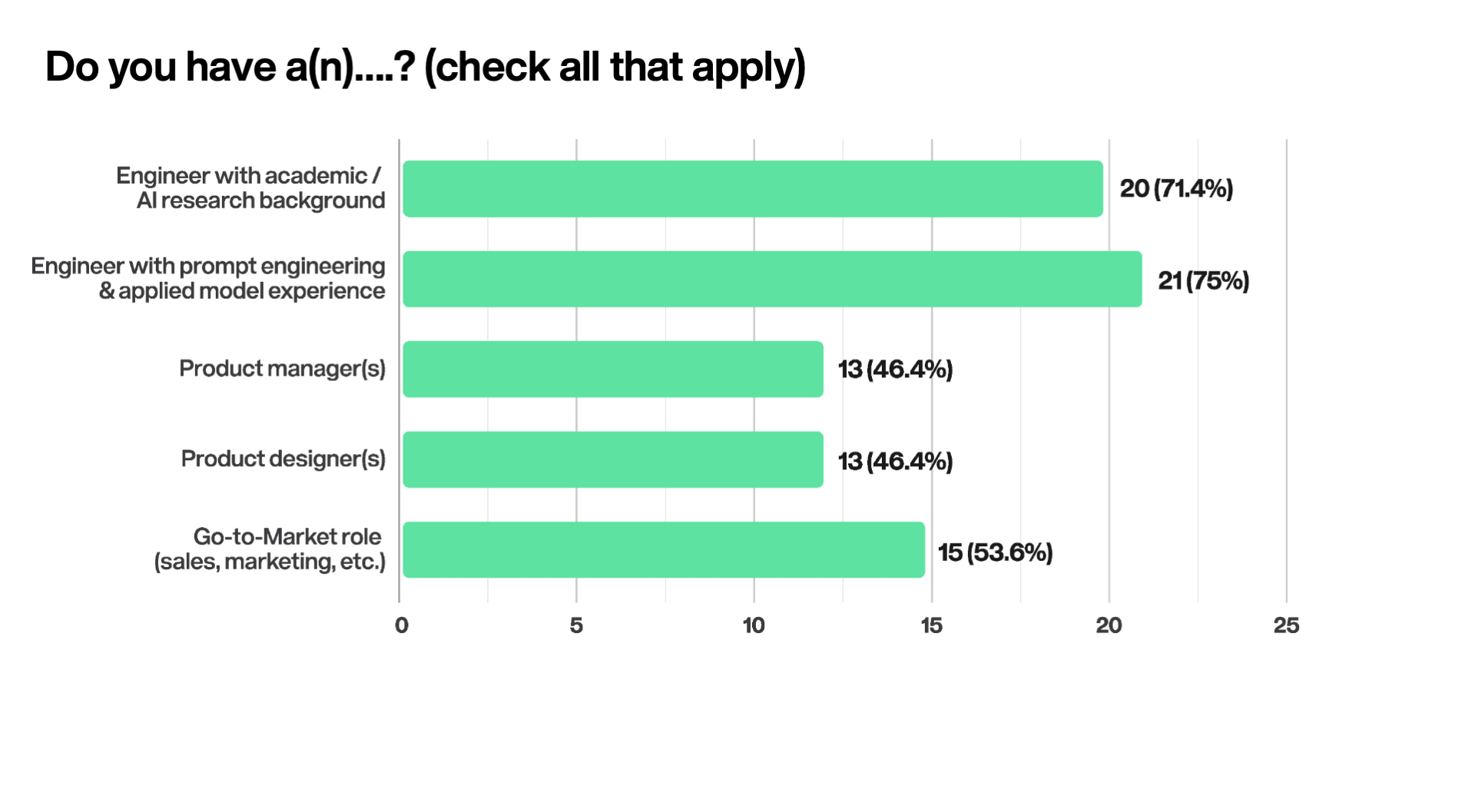
Is engineering talent really that hard to find?
When asked about the toughest hires:
- 43% mention technical talent
- 30% specify AI/ML talent
There is often an assumption that technical talent is the most challenging to hire, but the rest of respondents either reported that they didn’t have any challenges around hiring, or showed more diversity in the roles they struggled to fill — such as UX, product design, and sales.
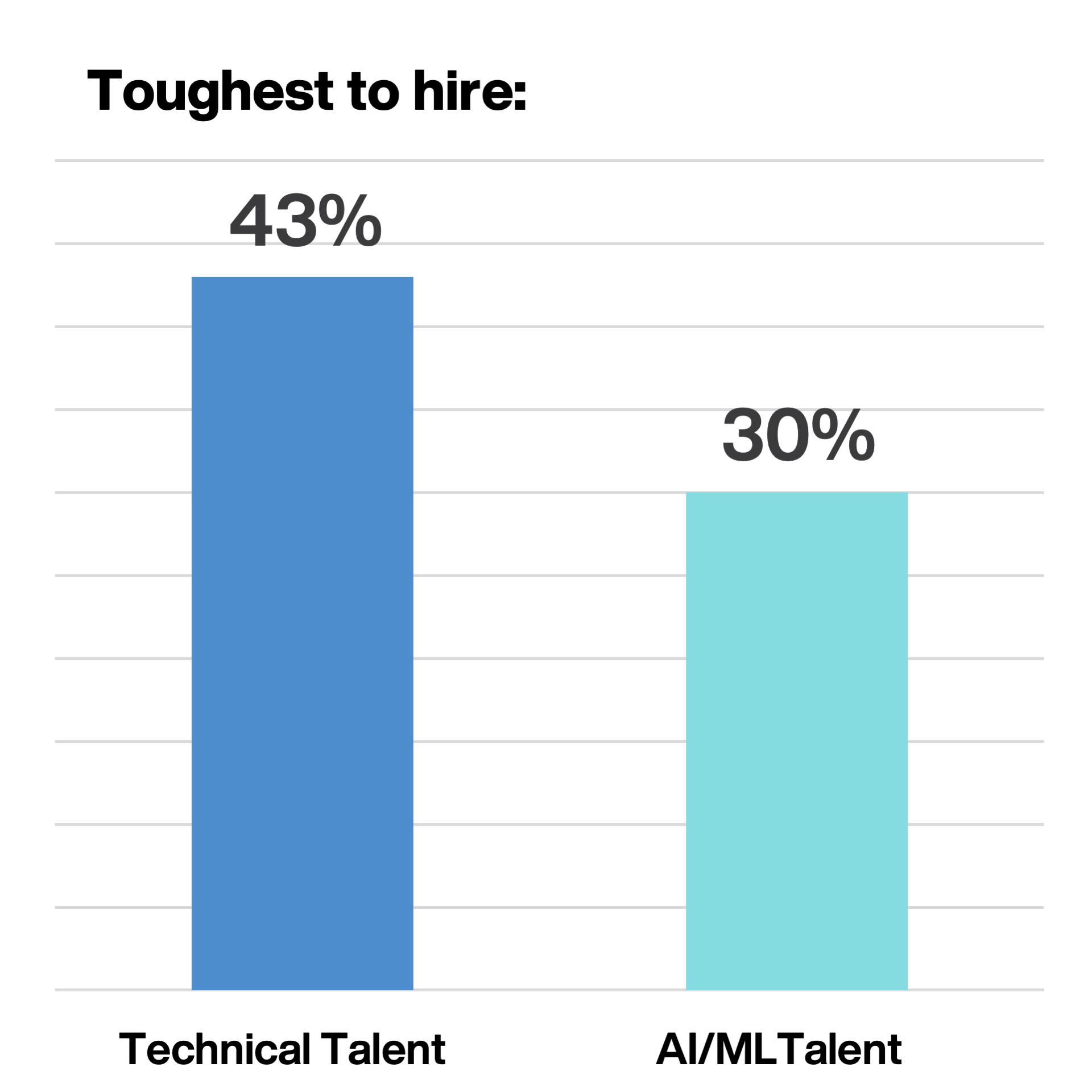
“Engineers who are both strong technically and have a strong Product + UX instinct. This is especially important to us because we have a very non-technical ICP. Engineers often struggle to build products for non-engineers.” —Cassidy
“Product Designer.” —Stealth Startup
“*Good* GTM.” —bem
“Sales leaders with both AI and pharma experience on the business side, versus the IT side.” —Sorcero
“Finding the right technical lead has been a bit harder than I anticipated. Ideally, they’d be a strong technical lead, enmeshed in AI for the past year or two, as well as deeply passionate and self-motivated to learn, build, and iterate using new AI tools — with concrete evidence they’ve personally done a bunch of experimentation themselves. Finding the combination of those, with actual experience in the latter, is harder to find at the moment.” —Ichi
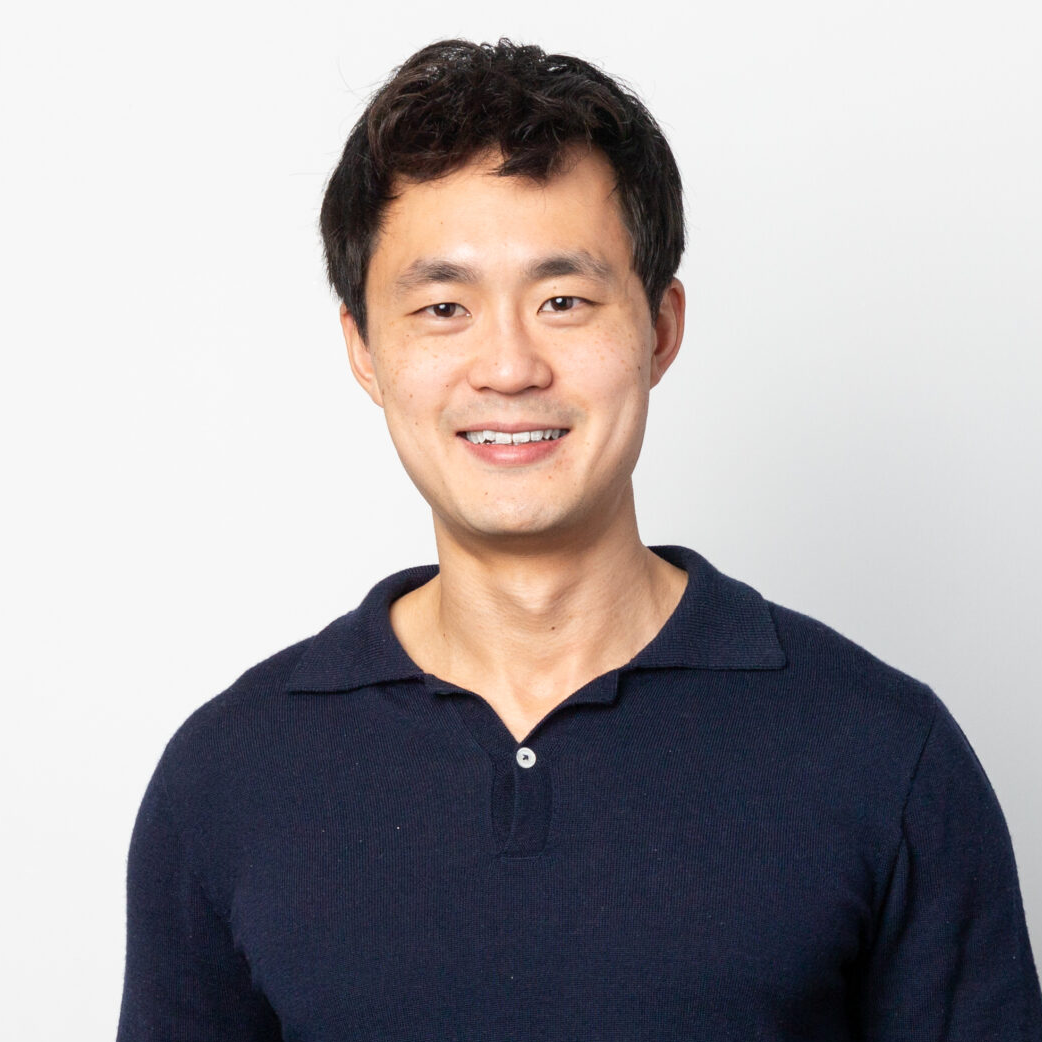
Investor Insight: Tony Liu
“This is about as much challenge as we’d expect to see in hiring strong technical talent. But it was surprising and reassuring to see how many were investing in GTM hires early. Many companies don’t invest in this early enough.”
Technology
How founders use models, data and tools to build AI products
The founders surveyed display a wide range of approaches to how they select, fine-tune, and leverage the latest foundation models — or not.
What foundation models are most popular?
The easy answer is OpenAI’s GPT. But the reality is more complicated — and varies by growth stage. GPT is ubiquitous, but building on multiple foundation models is almost as prevalent:
- 89% use at least one GPT version
(with 80% using GPT-4o) - 75% use more than one model
- 54% use 4 or more models
As startups mature, they’re more likely to diversify beyond GPT — 80% of Series A and later use alternate models, compared to 60% of pre-seed and seed stage. Gemini, Llama, and Claude are the most popular models after GPT. But there’s an interesting caveat: pre-seed and seed stage companies are more than twice as likely to use Mistral compared to Series A and beyond. When diving into what would prompt founders to switch from using GPT as their primary foundation model, 75% mention cost and/or performance. However, respondents share a wide range of opinions on what would really motivate them to transition.
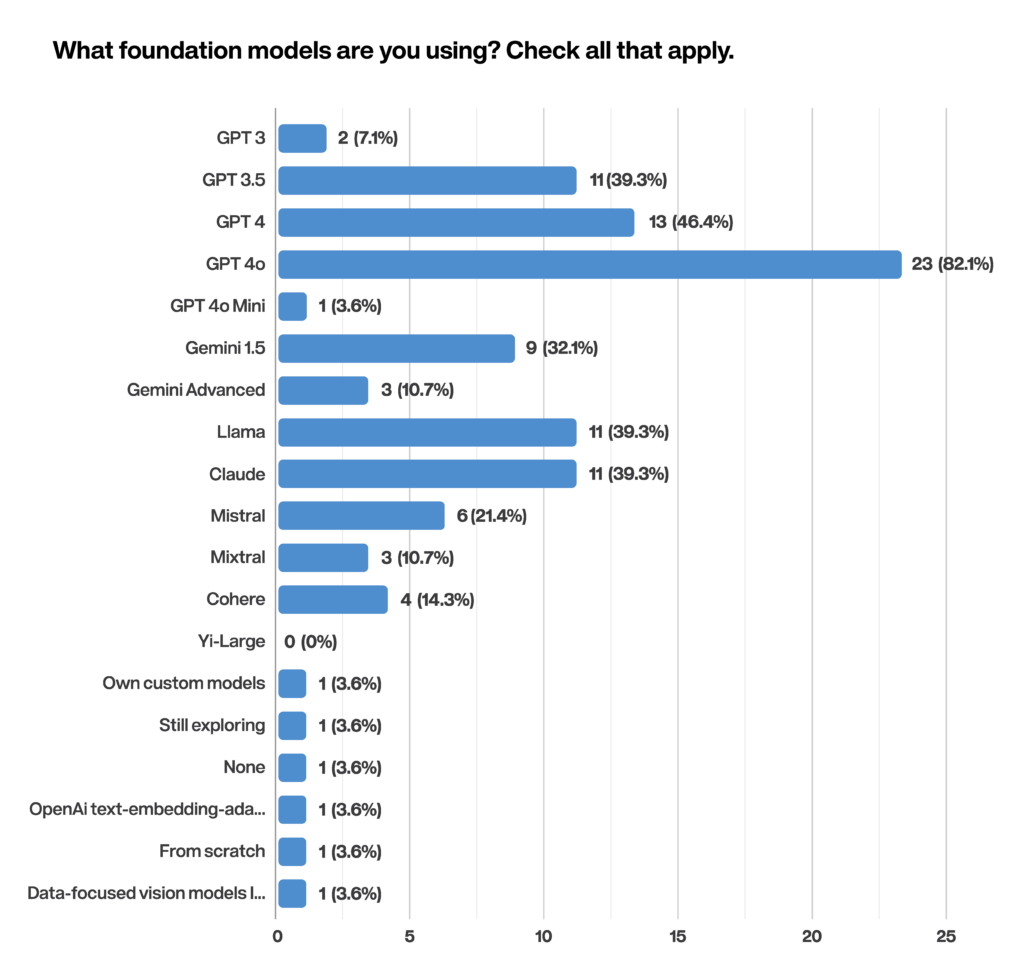
“We continually compare models on both performance and cost, and are willing to switch if we can get as-good performance at a cheaper cost.” —Vannevar Labs
“Quality and latency, more than cost.”—Mindtrip
“Better coding capabilities.”—Purgo AI
“Performance and value are the biggest factors for us using AI. Low latency only matters for some specific use cases that we would like to pursue.”—Hona
“We expect models to get better and better, and cheaper and faster, quickly.”—Stealth Startup
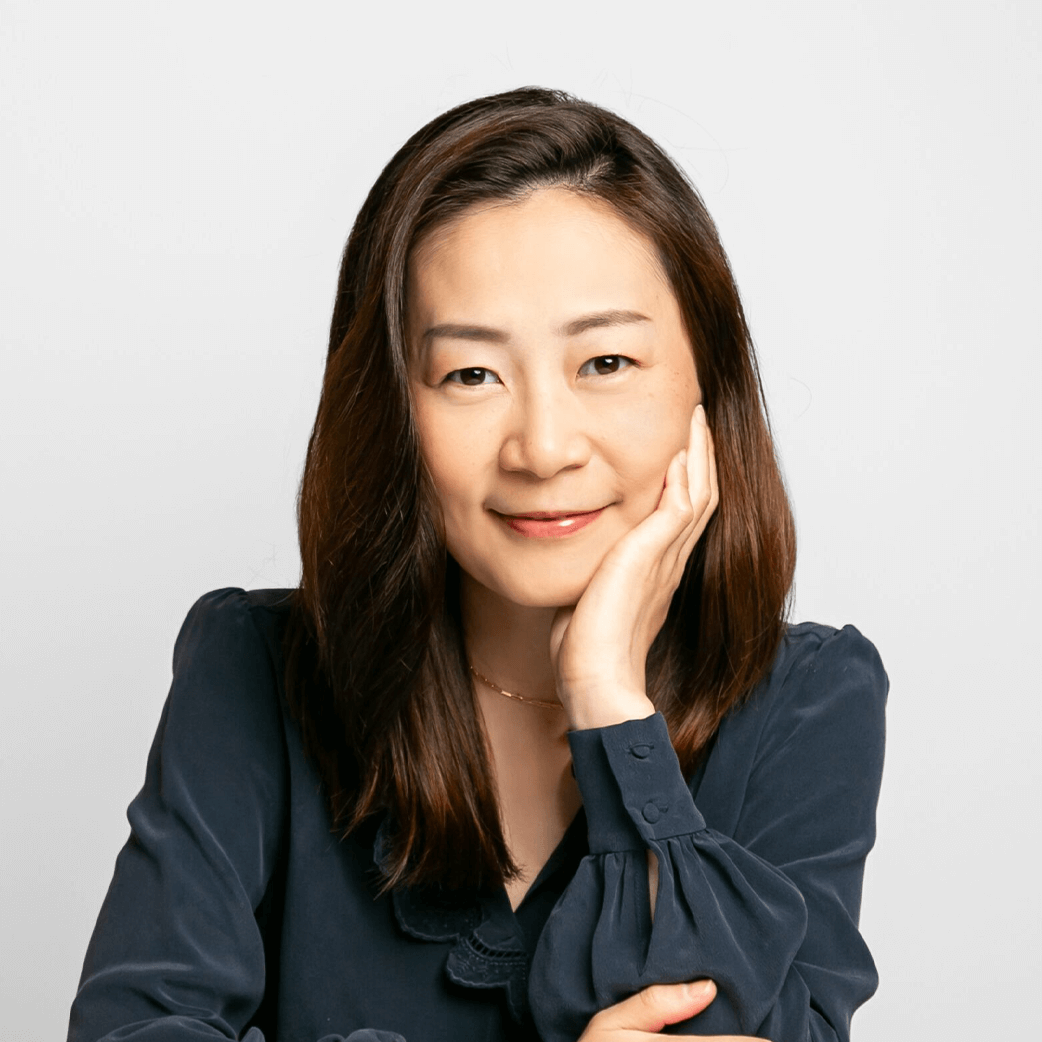
Investor Insight: Rebecca Li
“The foundation model landscape is changing fast. On one hand, the converging trend on model performance has led to people choosing a variety of models; on the other hand, lots of people still choose the top 3 shops as a more future-proof option as the state of the frontier keeps moving forward.”
How do founders choose models?
Founders cite similar reasons for choosing a model as they do for switching from GPT to an alternative: cost, performance, ease of use, and accuracy.
However, repeat founders are nearly twice as likely as first-time founders to mention cost as a chief consideration (44% vs. 25%).

“We started with OpenAI models as they were the early leader. We are increasingly experimenting with Claude models because our customers are asking for VPC deployments, and Claude can be deployed inside a customer account.” —Delphina
“Easy API and cheap to deploy for testing ideas at a currently low user volume. Good enough for our relatively simple purposes.” —Maven
“Ease of deployment and usage from Azure OpenAI. General robustness and capability of
4o model above other models.” —Purgo AI
How common is model fine-tuning?
Building an entirely new model involves deep work and is exceedingly rare, with only one founder reporting they are exclusively building their own model from scratch.
But roughly half of startups are fine-tuning models — training an off-the-shelf model on new data for specific use cases. Of those, 70% are creating products for consumers or vertical SaaS.
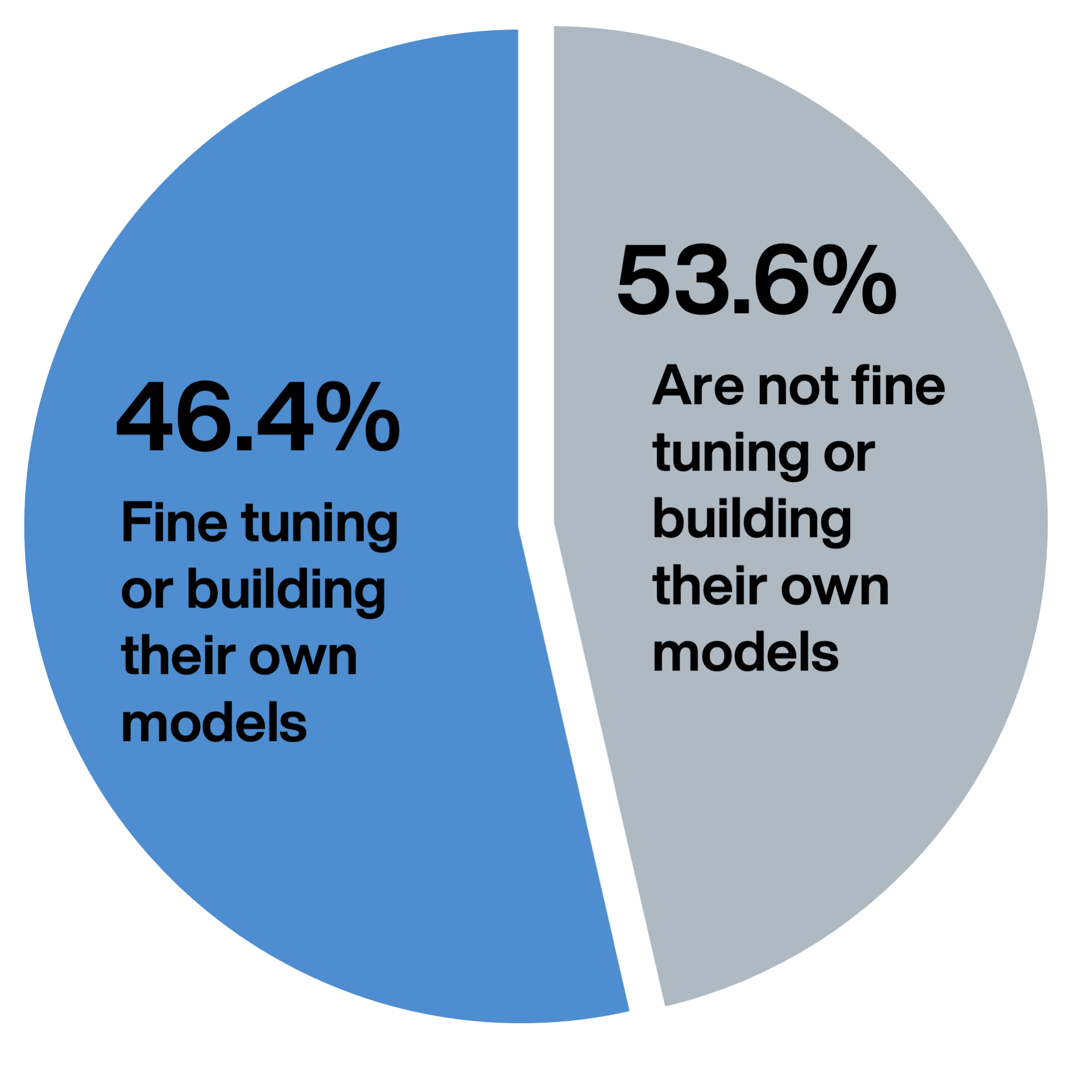
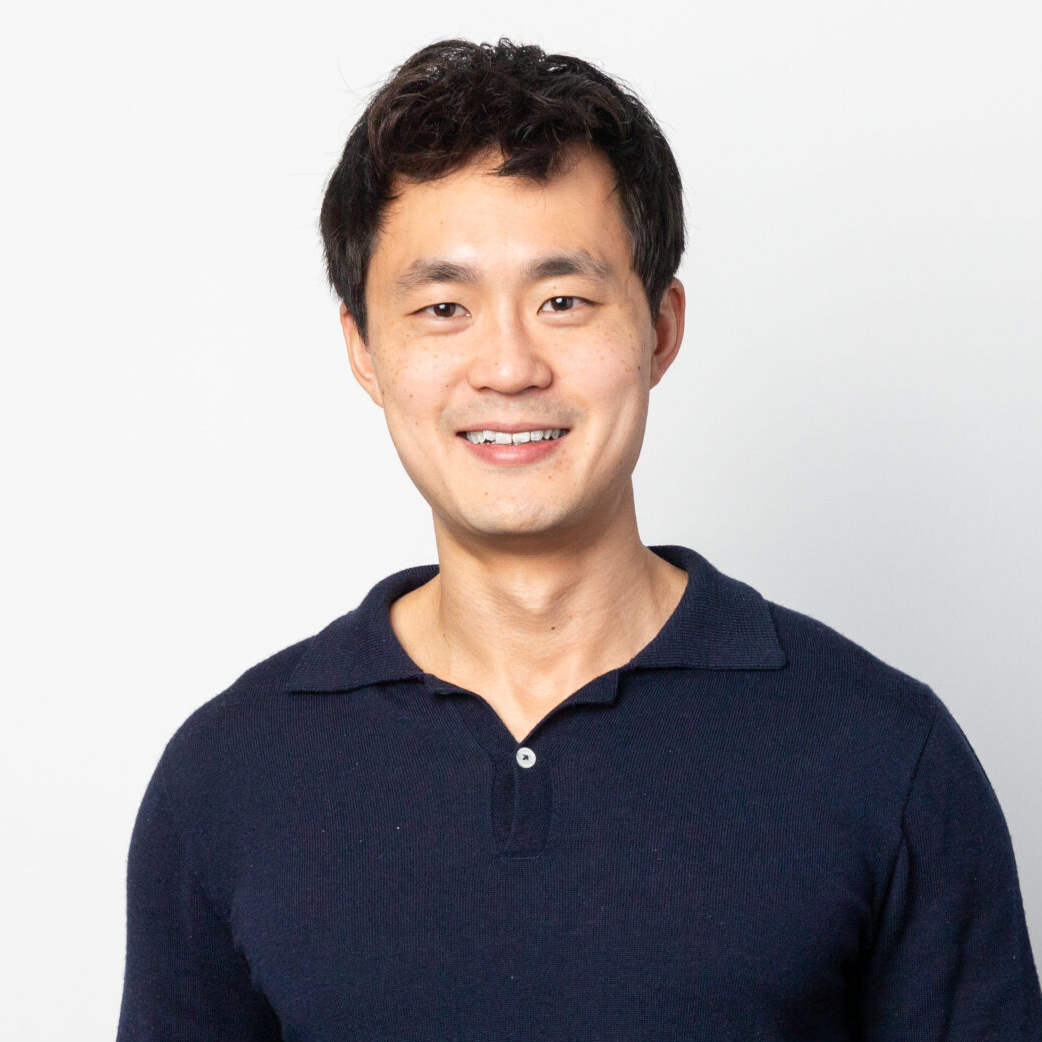
Investor Insight: Tony Liu
“Choosing RAG vs. fine-tuning is still an evolving conversation. In fact, it’s probably not choosing one or the other but figuring what is relevant for a specific use case. How companies choose what they do to refine models, improve latency, and reduce cost will be more interesting to watch over time, especially as new capabilities emerge in frontier models.”
What data sources are most often used for training?
Most startups are leveraging proprietary data (61%) or public datasets (54%) to train their models.
But the likelihood of using synthetic or third-party data varies by company stage:
- 55% Series A or later
- 19% Pre-Seed or Seed
Still, there are a few companies that don’t train their models, and instead implement them off-the-shelf.
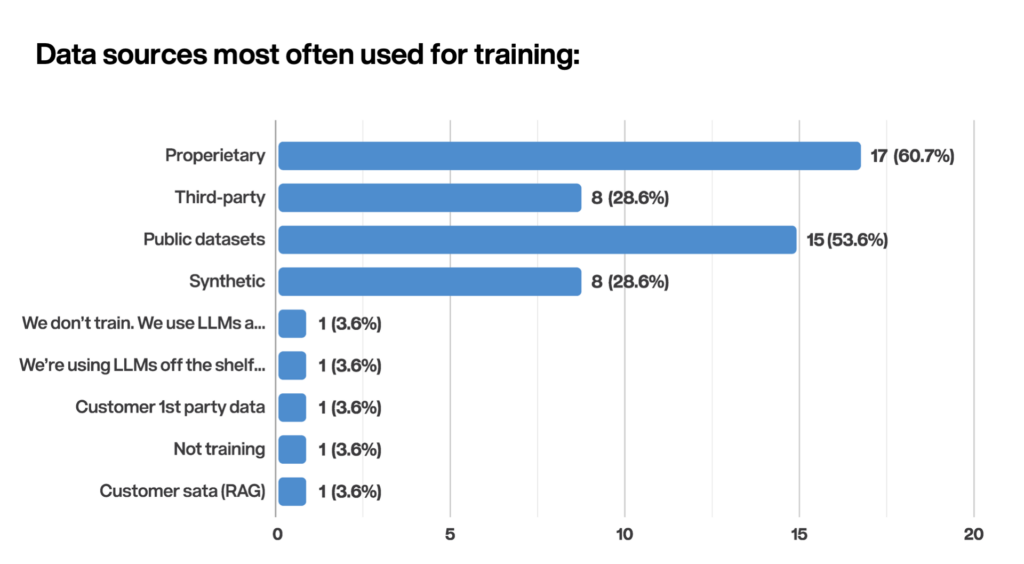
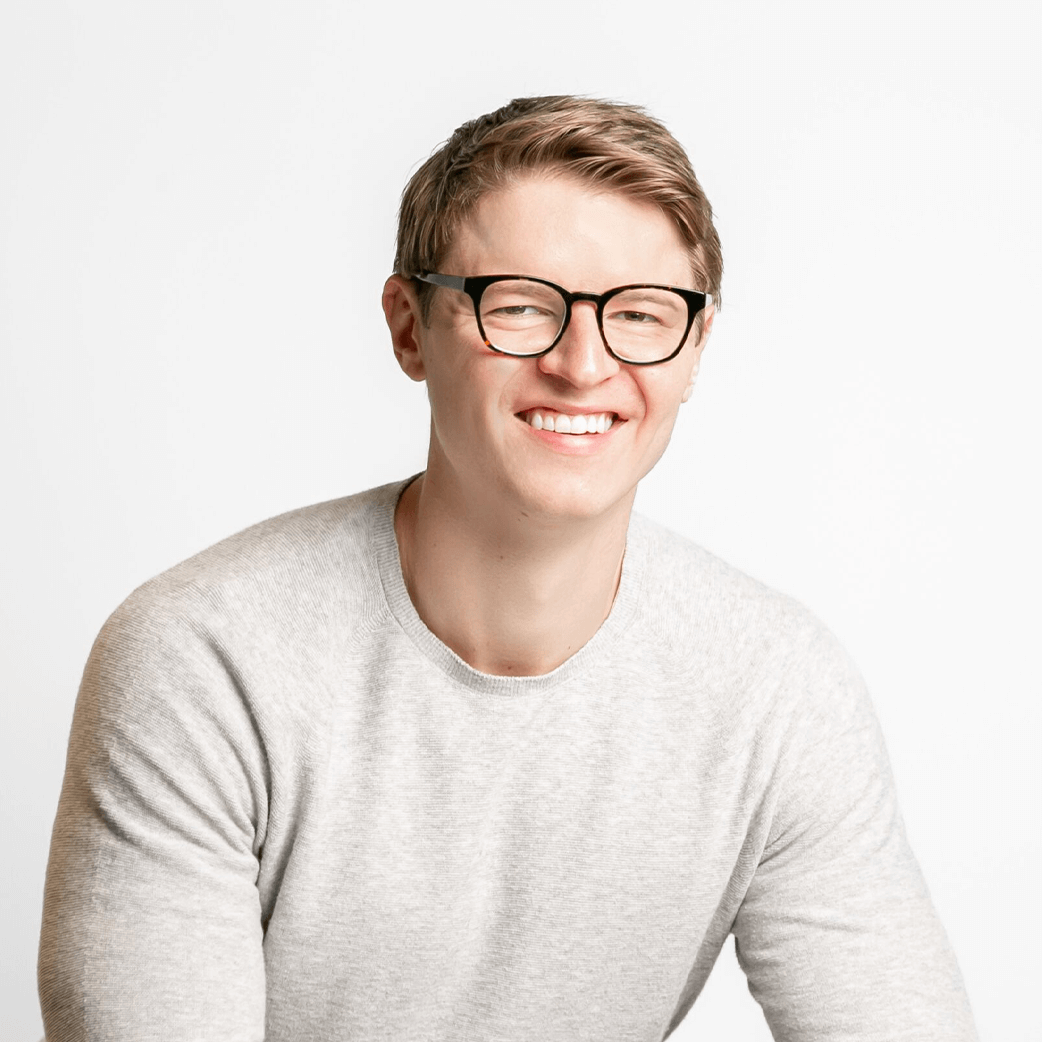
Investor Insight: John Cowgill
“Even with LLMs trained on internet scale data, companies with a proprietary data asset are still in the best position to have a long term moat. Any company that isn’t thinking about how they can cultivate a unique data asset over time will get left behind.”
How are multimodal tools impacting applications?
Multimodal capabilities describe a model’s ability to cover multiple “modalities,” like text, video, or image — but we’re still in the infancy of adoption.
Only 30% of founders surveyed are using multimodal capabilities in their products, with adoption rates varying by sector:
- 40% of consumer and vertical SaaS applications
- 28% of developer tools or infrastructure
“AgentOps supports tracking for multimodal AI agents that use images and screenshots.”
—AgentOps
“We are able to interpret graphical information and ingest images from the factory line.” —Sygma
“Ability to interact with pictures and charts versus just text, given GPT-4o’s multimodal abilities.”—VP of Finance & BizOps, Glean
“Made ingest of screenshots, receipts, and email content much easier. Voice will be big. As we work on our On Trip iOS app, starting with a photo/GPS combination is interesting.”—Mindtrip
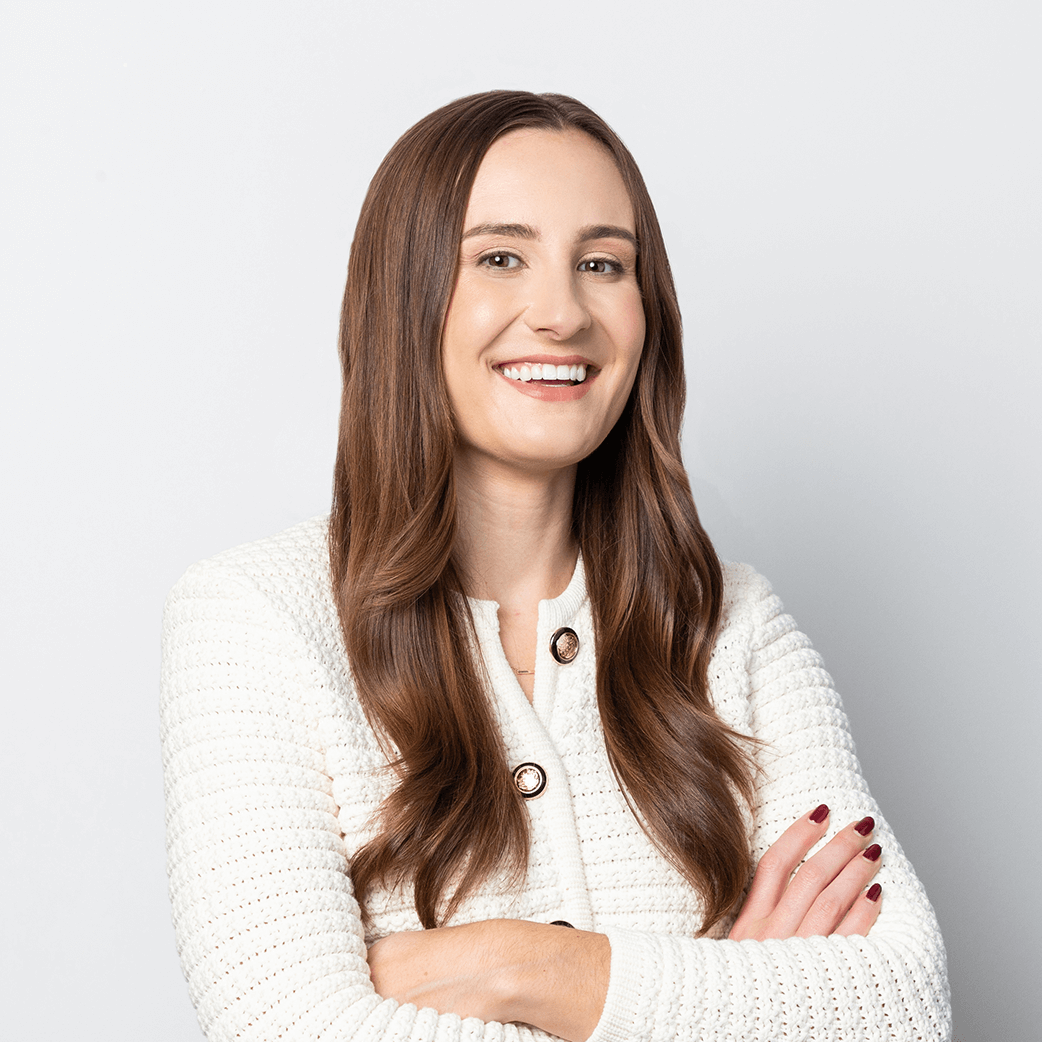
Investor Insight: Amy Cheetham
“I really see this changing more, especially as it applies to voice and vertical SaaS applications. I’m already seeing companies think differently about the type of customer experience you can create by incorporating these new-found capabilities.”
Which AI tools are the most valuable to founders?
Just shy of 90% of respondents are using some type of AI tooling either to build their platform or to enhance their own work, with the majority of tools supporting infrastructure and MLOps.
Which AI-related tools do you find most valuable?
“LangChain, as buggy as it is, is useful for providing one common interface for lots of different model providers/APIs.” — Cassidy
“There are so many out-of-the-box tools that are great to expedite prototyping (and sometimes production tools), such as LangChain and Pinecone. That said, broadly speaking, at this stage any tool using AI is helpful — because having our teams use these tools helps us all be more empathetic and curious to the current capabilities of AI. That creates valuable insights for how to build better products using these tools.” —Ichi
“LangSmith solves a real problem and pain we have around monitoring, regression and latency, and cost monitoring.” —Seed Startup
“LanceDB — cheap, scalable vector store that makes experimentation simple.” —Dosu
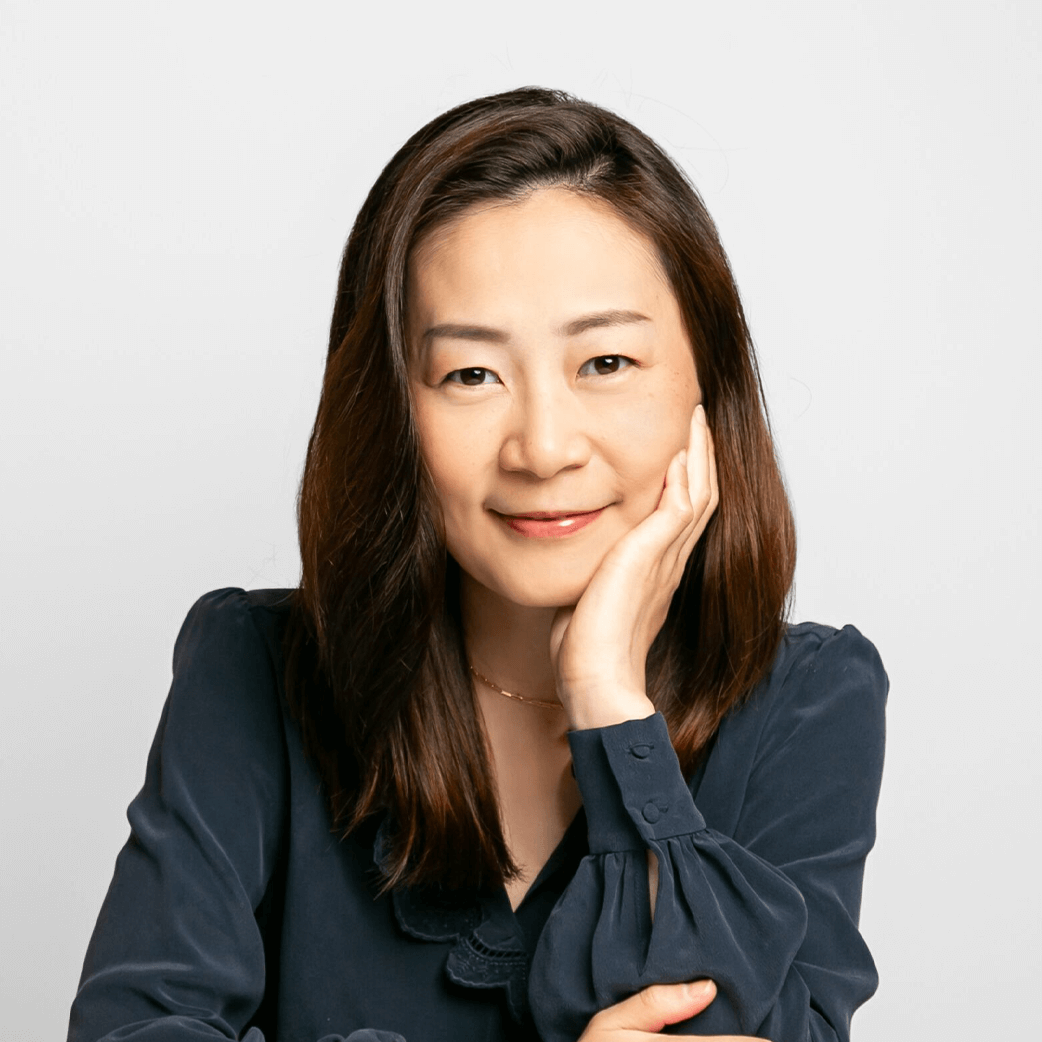
Investor Insight: Rebecca Li
“80% of these tools are closed vs. open source. That’s not surprising at this stage, but I wonder how much that will change over the coming years and if that represents more opportunity.”
Challenges
What keeps founders up at night?
When asked about their challenges, startup founders tended to cite predictable pain points (such as talent and product-market fit) while unexpectedly dismissing others (like defensibility).
What are the biggest challenges in building a company?
These founders give equal weight to acquiring customers and talent when it comes to naming their challenges.
- Only 16% of pre-seed and seed founders mention talent as a major challenge
- But of those who did mention talent, 80% are repeat founders
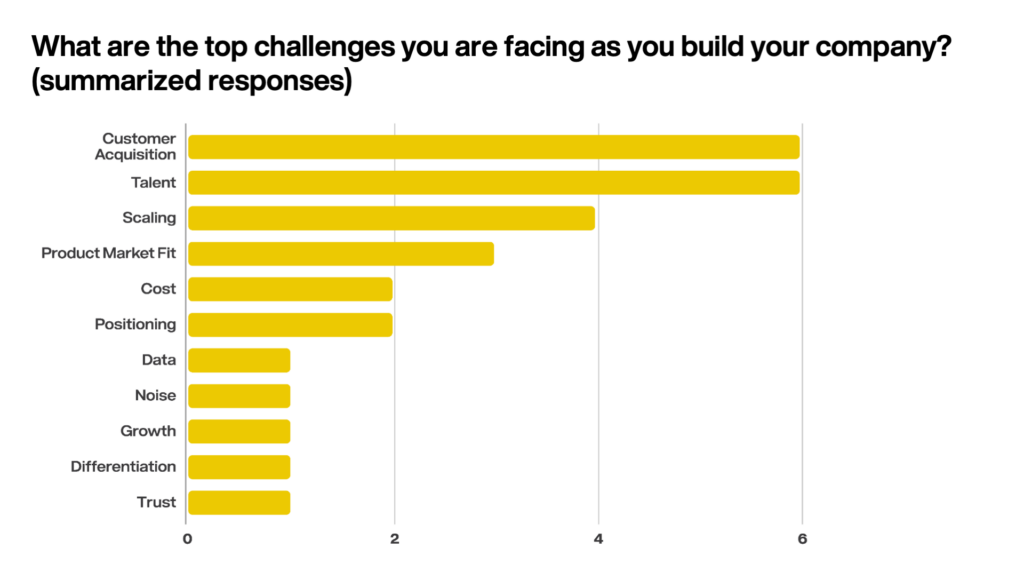
“Trust. There is an increased scrutiny and skepticism of AI outputs. How do we start delivering value immediately while building trust and growing scope over time?” —Dosu
“Positioning, selling, and implementing an AI-native product to a legacy industry with buyers and users who are not traditional software users.” —Sorcero
“Cost of GPU and fast developments in AI.” —Puppydog.io
“The fundamental concepts of GenAI are relatively easy to understand. However, given a) the newness of the space; and b) the speed of innovation — the notion of a ‘well worn path’ largely doesn’t exist. There isn’t a ton of prior art to learn from for how to build a great vertical-AI solution. This is a challenge, but also exciting, as it indicates that the potential impact with relatively small innovations could be huge. What this says to me is that focusing on first principles for how to build will be far more important than choosing the best path, as best-likely doesn’t exist. Rather, it will be more about being able to adapt to what will be most beneficial at any given point in time within a given context.” —Ichi
“We are in the GTM phase, but still early — building an audience and distribution. Some minor challenges with how AI and traditional models coexist, but that’s more of an opportunity.” —Mindtrip
“Hiring engineers, getting AEs productive, and scaling!” —VP of Finance & BizOps, Glean
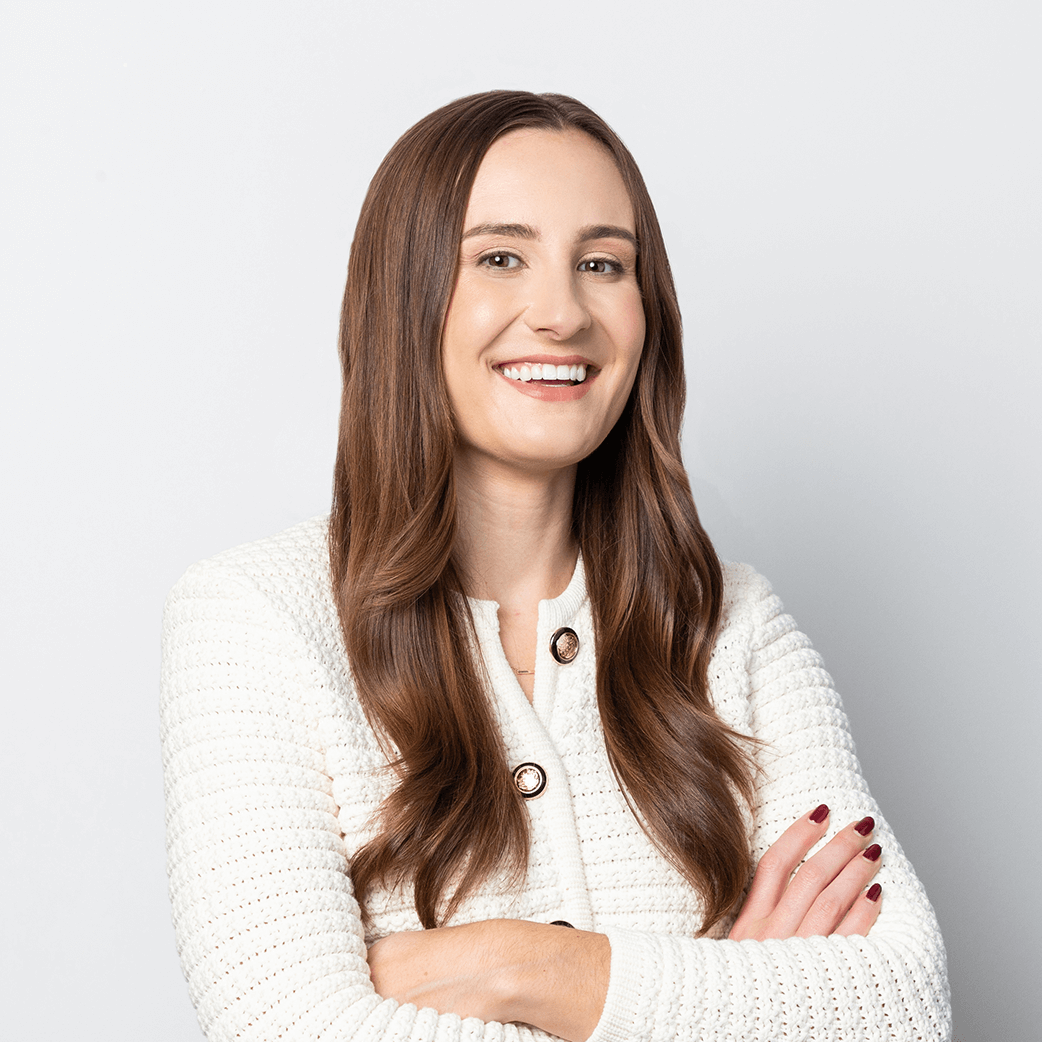
Investor Reflection: Amy Cheetham
“The core challenges of early stage startups are the same whether you’re an AI-native startup or not. These problems have been the same for decades, but now we’re adding a layer of complexity as teams learn how to harness the power of AI.”
How are founders dealing with model regression?
Models are constantly updated, making model regression a unique yet increasingly important issue for AI startups. If a new version of a model performs worse on relevant tasks than its predecessor, it could introduce unexpected changes in their product’s behavior. While some founders seem lost on where to begin, the majority (60%) are already making an effort to keep up with model regression.
A few mention Kubeflow or Langsmith, but most respondents addressing model regression are doing so through their own manual setups:
“Read Twitter and try it out.” —Million.dev
“We’ve built our own evaluation frameworks and pipelines to continuously test our automated fine-tuning process.” —bem
“MLOps has a system to monitor and correct as required. We also have the ability to hot swap models in case of regressions.” —Sorcero
“Hard to do this!” —Aisop’s Fable
“We typically constrain our use cases such that the underlying data distribution doesn’t change very much, and we pin our model versions so the behavior doesn’t change without us knowing. Cases where we’ve experienced performance regression have been when we’ve fine-tuned our own LLMs on domain-specific tasks.” —Vannevar Labs
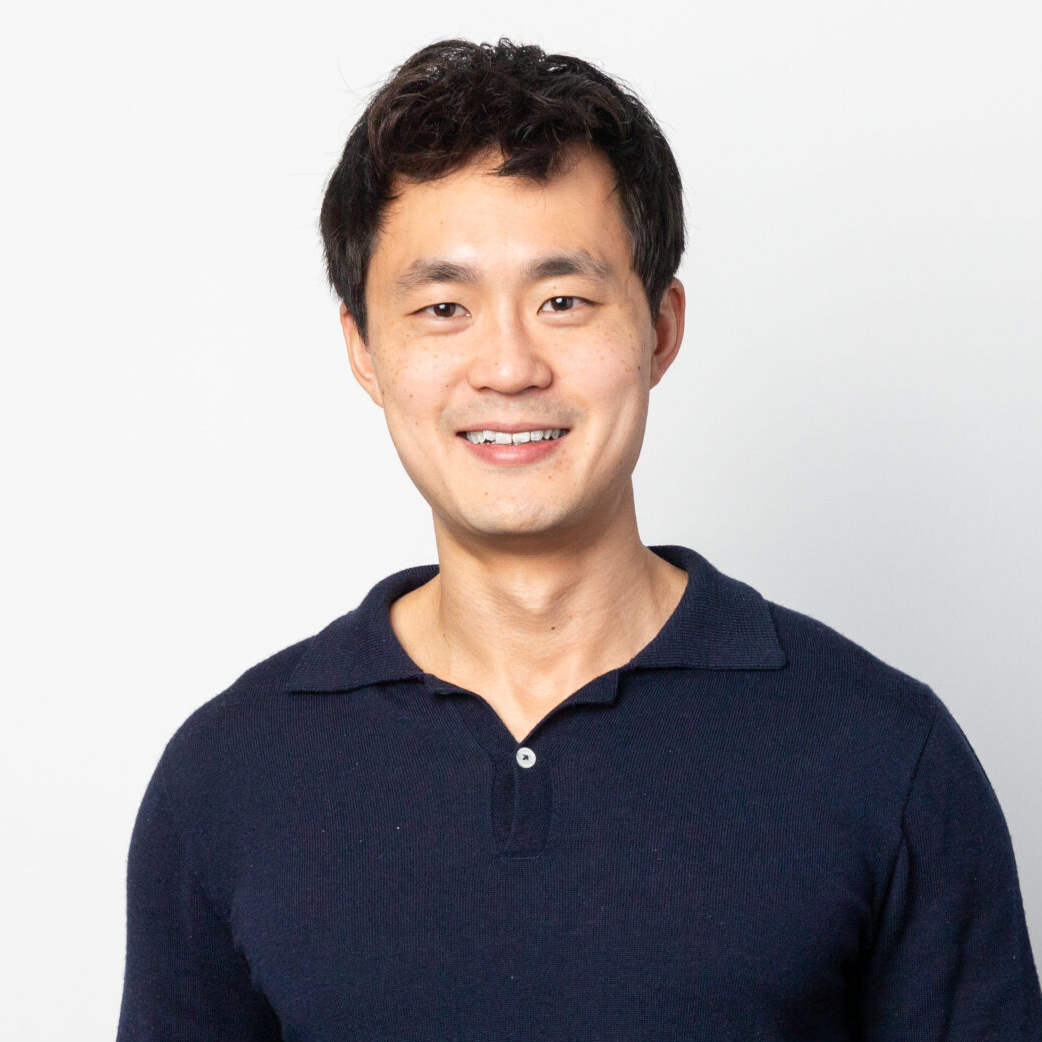
Investor Insight: Tony Liu
“‘Read Twitter and try it out’ was such an honest answer. And so GenZ. But it’s spot on and a smart way to learn fast.”
Do founders worry about defensibility?
Sam Altman already warned that OpenAI would “steamroll” AI startups, but founders do not seem worried about defensibility (the possibility of what they have built becoming part of a foundational model over time).
Only 13% expressed even ‘slight concern’ about defensibility.
Rather, most founders voiced confidence that their products delivered unique value or had such a niche purpose that it wouldn’t make sense for foundation models to absorb.
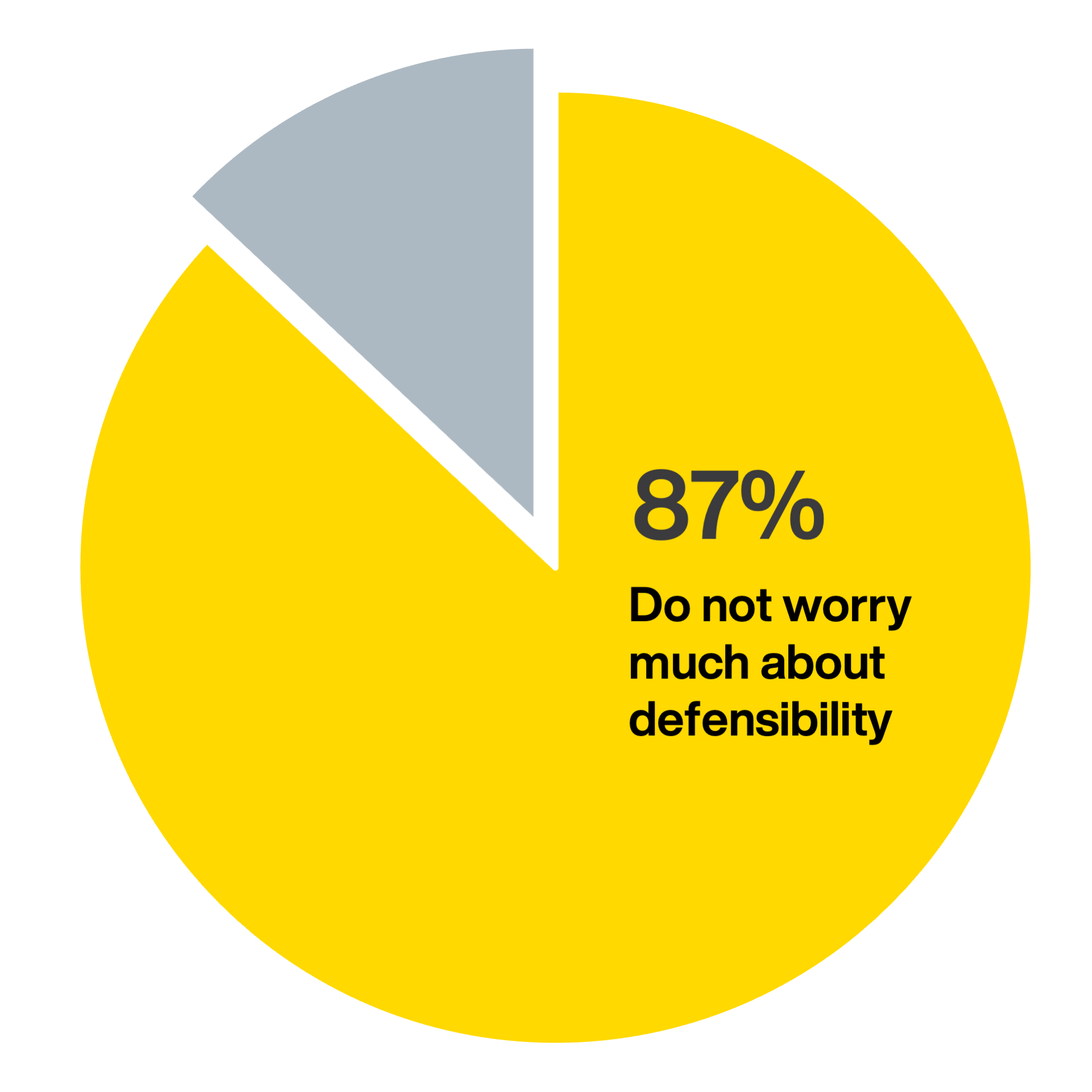
“I worry about it some because that is my job. But I think the industry we’re in, the problem we’re solving, isn’t as likely to be fundamentally disrupted by foundation models, at least not for years.” —Seed Startup
“Not very worried for our niche, because our users are a more important aspect of our experience than our AI. But defensibility is clearly a major issue across the industry in general.” —Maven
“We try to build with the advancement of these models in mind. When we build a product, we try to build something that would only be made more useful by better foundation models.” —Cassidy
“We picked a space where we believe vertical AI will beat horizontal.”—Mindtrip
“Not worried. Our IP is in our data structures and architecture. Better models make our job easier.” —System Two Security
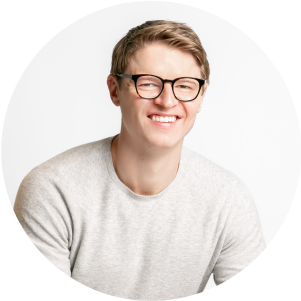
Investor Insight: John Cowgill
“That founders have so few concerns about defensibility surprised me the most – but maybe shouldn’t have. You have to have impossible belief in your idea as a founder, or why else would you do it?”
What’s the most painful part of building in AI right now?
When asked about the difficulties of building in AI at the current moment, a few common themes emerged across all founders’ open-ended responses. First time versus experienced, early or later stages, most respondents mention:
- Rapid pace of change
- Lack of quality tooling
- Inability to access data
- Uncertainty

“The ground is shifting under everyone’s feet, and it’s not clear where we’ll be in six months. It makes it hard to fully commit to specific strategies with a small team.” —Sygma
“Managing the entire process/workflow is tough. All the products out there to do so are severely lacking in simplicity. Also training models is hard today, since you typically need multiple nodes and many GPUs.” —Focal
“Lots of noise in the market. Market is moving extremely fast.”
—VP of Finance & BizOps, Glean
“Too much hype around AI products that don’t actually work translates to skepticism for the whole industry. We don’t use AI in any of our branding so customers focus on the functionality and outcomes.”
—bem
“Lack of regression testing tools and other standard software quality building blocks.”—Sixfold AI
“Buying data.” —Stealth Startup
“Uncertainty around what the future looks like, given how fast the space is moving. Realistically this is an advantage as a startup, since we’re able to adapt quickly and take advantage of unforeseen shifts — but it can make it hard to plan long-term.” —Cassidy
Key Takeaways
As a whole, the survey reveals a diversity of perspectives—in other words, there is no formula to build an AI startup. But for other founders and entrepreneurs, there are valuable learnings to use as you build your own business:
Calibrate yourself. Are you exploring the landscape of models, or are you potentially missing out? Are you leveraging your own real-world data sets for training? Will adopting multimodal capabilities give you a competitive edge?
Make decisions with conviction. From where you choose to headquarter your company to how you approach defensibility, being a founder is all about making choices. It takes courage to start a business, and part of that means making decisions that may be wrong.
Celebrate your place in this historic moment. There are naysayers all around who only see the risk or negative applications of AI. But founders who are building companies right now are optimistic — and so are we. There’s enormous potential for AI to make our jobs better and our future brighter, and you’re part of it.